In causal discovery, the aim is to uncover the underlying causal mechanisms that drive the relationships between a collection of observed variables. It is a research topic with applications in many areas, including medicine, biology, economics, and social sciences. In principle, identifying causal relationships requires interventions (a.k.a. experiments). However, this is often impossible, impractical, or unethical, which has stimulated much research on causal discovery from purely observational data or mixed observational-interventional data. In this talk, after surveying the causal discovery field, I will discuss some recent advances, namely on causal discovery from data with latent interventions and on the quintessential causal discovery problem: distinguishing cause from effect on a pair of dependent variables.
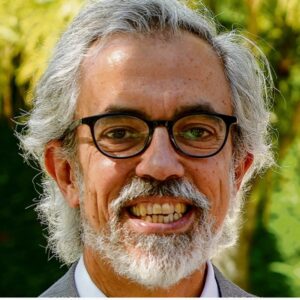
Mário Figueiredo received his PhD (1994) in Electrical and Computer Engineering from Instituto Superior Técnico, University of Lisbon, where he is an IST Distinguished Professor and holder of the Feedzai Chair on Machine Learning. He is a senior researcher and group leader at Instituto de Telecomunicações. His research areas include machine learning, signal processing, and optimization. He received several honors and awards, namely: Fellow of the Institute of Electrical and Electronics Engineers (IEEE), Fellow of the International Association for Pattern Recognition (IAPR), Fellow of the European Association for Signal Processing (EURASIP), W. R. G. Baker Award (IEEE), EURASIP Technical Achievement Award, member of the Portuguese Academy of Engineering, member of the Lisbon Academy of Science.