This talk will describe a journey that took me from a world in which shades of uncertainty were deemed critical to data analysis and decision making to another world, in which shades of uncertainty are viewed as consequences of cause effect relationships that govern our understanding of the world. After summarizing some glaring deficiencies of data fitting methods, I will contrast them with model-based causal inference approaches and demonstrate how the latter can achieve a state of knowledge we can call “Deep Understanding,” that is, the capacity to answer questions of three types: predictions, interventions and counterfactuals. I will further describe a computational model that facilitates reasoning at these three levels, and demonstrate how features normally associated with “understanding” follow from this model. These include generating explanations, generalizing across domains, integrating data from several sources, assigning credit and blame, recovering from missing data, and more. I will conclude by describing future research directions, including automated scientific explorations and personalized decision-making.
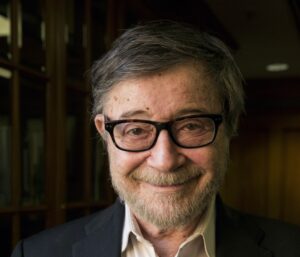
Judea Pearl is Chancellor professor of computer science and statistics at UCLA, where he directs the Cognitive Systems Laboratory and conducts research in artificial intelligence, human cognition, and philosophy of science.
He has authored three fundamental books, Heuristics (1983), Probabilistic Reasoning (1988) and Causality (2000, 2009) which won of the London School of Economics Lakatos Award in 2002. More recently, he co-authored Causal Inference in Statistics (2016, with M. Glymour and N. Jewell) and “The Book of Why” (2018, with Dana Mackenzie) which brings causal analysis to a general audience.
Pearl is a member of the National Academy of Sciences the National Academy of Engineering, a Fellow of the Cognitive Science Society the Royal Statistical Society and the Association for the Advancement of Artificial Intelligence. In 2012, he won the Technion’s Harvey Prize and the ACM Alan Turing Award “for fundamental contribution to artificial intelligence through the development of a calculus for probabilistic and causal reasoning.” In 2022 he won the BBVA Frontiers of Knowledge Award for “laying the foundations of modern artificial intelligence, so computer systems can process uncertainty and relate causes to effects.”