People often explain outcomes by imagining how they could have turned out differently, if some other aspects of the situation had been different. The mental representations and cognitive processes that underlie the construction of these counterfactual explanations differ in important ways from other sorts of explanations, such as causal ones. I illustrate some implications of these differences for eXplainable AI (XAI) by discussing three recent experimental discoveries. First I outline recent findings that people’s preferences for counterfactual explanations of an AI’s decisions do not fully align with the accuracy of their predictions of an AI’s decisions. Next I describe how counterfactual explanations of an AI’s decisions can influence people’s choices of safe or risky options. Third, I sketch preferences for simple causal explanations for prediction compared to diagnosis. I discuss the potential role of experimental evidence from cognitive science in future developments of psychologically plausible automated explanations.
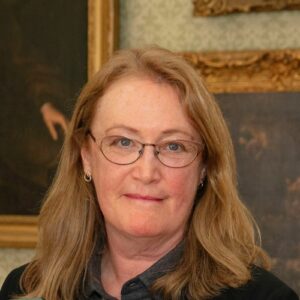
Ruth Byrne is the Professor of Cognitive Science at Trinity College Dublin, University of Dublin, Ireland, in the School of Psychology and the Institute of Neuroscience. Her research expertise is in the cognitive science of human thinking, including experimental and computational investigations of reasoning and imaginative thought, especially counterfactual thinking. Her books include “The rational imagination: how people create alternatives to reality” published by MIT press in 2005. A key component of her current research focuses on experimental investigations of human explanatory reasoning and the use of counterfactual explanations in eXplainable Artificial Intelligence.